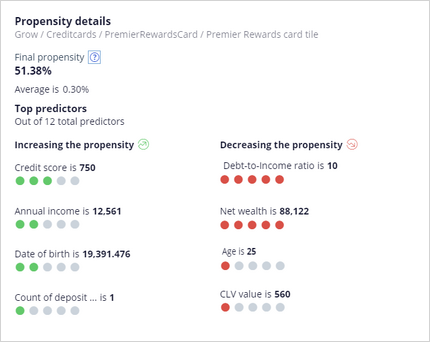
Explainable AI
U+ Bank uses Pega Customer Decision Hub™ to personalize the offers that customers see when they log in to the U+ Bank website. The adaptive models that calculate the propensity that a customer clicks on the offer are highly transparent. Customer Decision Hub includes tools that give local and global explanations for the adaptive model behavior.
Local explanations enhance the ability to understand the reasoning behind a single propensity calculation made by the model. Customer Profile Viewer offers local explanations of the decisions made by Customer Decision Hub, including the propensities calculated by the adaptive models that drive these decisions.
Global explanations refer to predictor importance in an adaptive model. Customer Decision Hub has built-in support to calculate predictor importance by using decision trees and random forests.
Video
Transcript
Local and global explanations support transparency in how predictive models calculate propensity. Local explanations enable understanding the rationale behind a single propensity calculation made by a predictive model, and they focus on understanding how the input predictors influence that single calculation. Global explanations focus on the overall behavior of a model and provide an understanding of which predictors are most important across all customers. Both local and global explanations are important to ensure that the models behave as expected and to build trust with customers and regulators in the use of AI.
U+ Bank uses Pega Customer Decision Hub to optimize customer interactions on the bank's website, and the Customer Profile Viewer report provides local explanations of the propensities calculated by the out-of-the-box adaptive models. You can review basic customer information for Joanna, including key indicators and demographic data. You can review recent interactions with Joanna in the timeline and how she responded to the decisions made by Customer Decision Hub. You can filter the interactions by the outcome and time frame.
Customer Profile Viewer also shows the current prioritization of next-best-action results. For the U+ Bank website channel, the direction is inbound, and TopOffers is the real-time container that connects with the website. Joanna is eligible for two credit card offers as determined by engagement policies and constraints. The adaptive models calculate the propensities for these offers. The final propensity used in the arbitration formula may differ from the model propensity, for example, when the customer is part of a model control group or when the adaptive model is still in the initial learning phase.
For example, U+ Bank can explain the decision to offer customer Joanna the offer with the highest propensity, the RewardsPlus credit card. The credit score and annual income predictors increase the propensity for Joanna to click on the personalized credit card offer. In contrast, the debt-to-income ratio and net wealth predictors decrease it. This explanation is valid for the RewardsPlus credit card offer to Joanna at this point in time.
In machine learning, predictor importance refers to the relative importance of each predictor in predicting the target variable. Customer Decision Hub includes built-in support for calculating predictor importance using decision trees and random forests. These techniques determine the relative contribution of each predictor to the predictions made by the adaptive model.
In Prediction Studio, the ADM predictor importance report lists the predictor importance score for each predictor, which indicates their relative importance in the model. By analyzing predictor importance, you can offer global explanations of the factors that drive the predictions made by the adaptive models. For example, when you select the Age predictor, you see that the importance of this predictor is relatively high in the adaptive model for the Premier Rewards credit card offer. You can export the report for further analysis.
You have reached the end of this video. You have learned:
- How Customer Profile Viewer provides data on past interactions with a customer.
- How Customer Profile Viewer provides local explanations for the decisions that Customer Decision Hub makes for a customer.
- How Prediction Studio supports global explanations of the factors that drive predictions.
This Topic is available in the following Modules:
If you are having problems with your training, please review the Pega Academy Support FAQs.
Want to help us improve this content?